Inferring Human Intentions from Predicted Action Probabilities
Lei Shi, Paul-Christian Bürkner, Andreas Bulling
Proc. Workshop on Theory of Mind in Human-AI Interaction at CHI 2024, pp. 1–7, 2024.
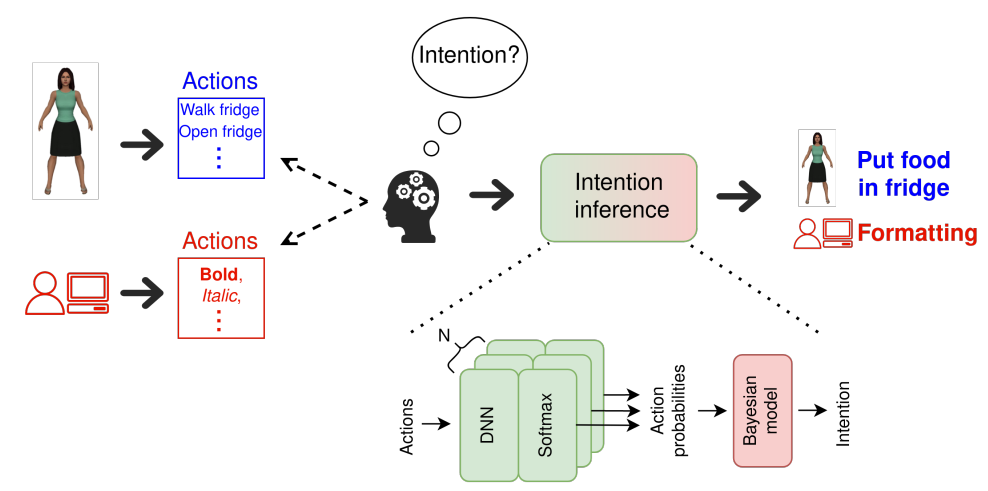
Abstract
Inferring human intentions is a core challenge in human-AI collab-oration but while Bayesian methods struggle with complex visual input, deep neural network (DNN) based methods do not provide uncertainty quantifications. In this work we combine both approaches for the first time and show that the predicted next action probabilities contain information that can be used to infer the underlying user intention. We propose a two-step approach to human intention prediction: While a DNN predicts the probabilities of the next action, MCMC-based Bayesian inference is used to infer the underlying intention from these predictions. This approach not only allows for the independent design of the DNN architecture but also the subsequently fast, design-independent inference of human intentions. We evaluate our method using a series of experiments on the Watch-And-Help (WAH) and a keyboard and mouse interaction dataset. Our results show that our approach can accurately predict human intentions from observed actions and the implicit information contained in next action probabilities. Furthermore, we show that our approach can predict the correct intention even if only a few actions have been observed.Links
Paper: shi24_chiw.pdf
BibTeX
@inproceedings{shi24_chiw,
author = {Shi, Lei and Bürkner, Paul-Christian and Bulling, Andreas},
title = {Inferring Human Intentions from Predicted Action Probabilities},
booktitle = {Proc. Workshop on Theory of Mind in Human-AI Interaction at CHI 2024},
year = {2024},
pages = {1--7},
doi = {}
}